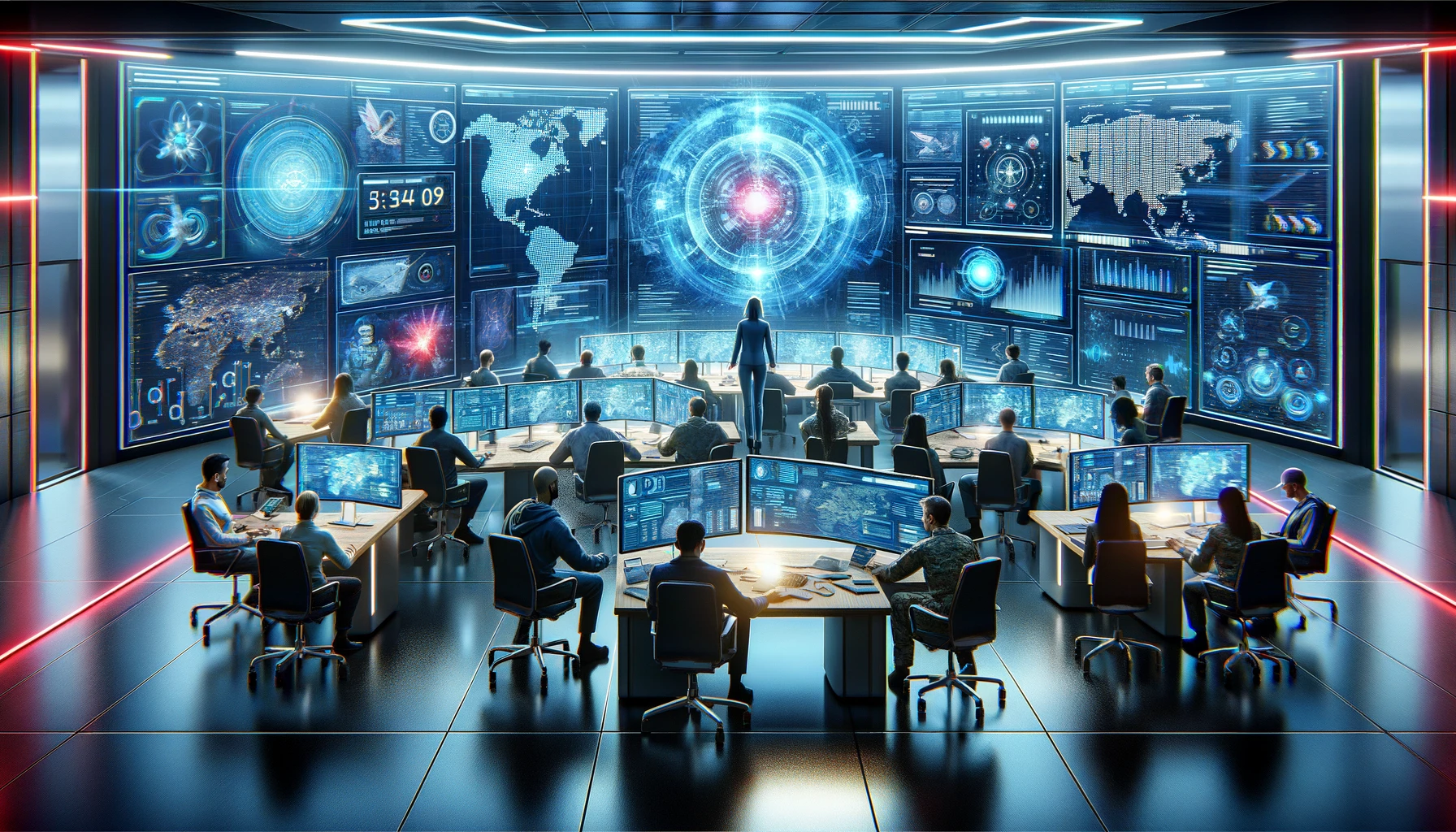
AI in Cybersecurity: Custom Solutions for Unique Challenges
With cyber threats continuously evolving, most organizations would agree that AI solutions can provide an extra layer of defense.
Luckily, AI systems can be highly customized to address the unique security challenges and risks facing different companies.
In this article, we’ll explore how businesses can leverage tailored AI tools to bolster their cybersecurity in alignment with their specific needs and vulnerabilities.
The Intersection of AI and Cybersecurity
This introductory section will provide an overview of how AI tools for cybersecurity offer unique and effective solutions to complex security challenges.
Understanding Cyber Threats and AI’s Role
Cyber threats are constantly evolving and becoming more sophisticated. Attackers use advanced techniques like social engineering, malware, zero-day exploits, and more to breach defenses. Traditional security solutions often struggle to keep up.
AI and machine learning have emerged as powerful ways to bolster cybersecurity:
-
AI can analyze massive volumes of data from networks and systems to detect subtle anomalies that indicate threats. This allows earlier threat detection compared to human analysis alone.
-
AI models can be trained on new types of attacks and adapts automatically. This enables more proactive defense against rapidly changing threats.
-
AI tools perform tedious tasks like reviewing system logs, freeing up security analysts to focus on higher value investigations.
Applying AI proactively is key for robust protection against modern cyberattacks.
AI Security Software: Beyond Traditional Measures
AI security software offers new advantages over traditional cybersecurity measures:
-
More comprehensive data collection and correlation – AI software gathers extensive system, network, and user data to establish fuller behavioral profiles. Advanced correlation detects threats that evade traditional tools.
-
Continuous self-tuning – AI algorithms continuously fine-tune threat models and adapt parameters autonomously to improve detection rates over time. This reduces reliance on human updates.
-
Lower false positives – By establishing accurate data baselines, AI tools minimize false alerts compared to rules-based systems with generic thresholds.
-
Simulated threat modeling – AI systems can simulate new attack scenarios and variations to existing threats. This allows testing and optimizing defenses before attacks occur.
Setting the Stage for Custom AI Implementations
While AI security software offers significant improvements, each organization faces unique threat landscapes, vulnerabilities, and risk appetites.
Custom AI solutions allow businesses to tailor cybersecurity capabilities to their specific environment and requirements. Key benefits include:
-
Models trained on the organization’s own network and usage data, maximizing relevance of threat detection.
-
Close integration with existing security stacks, minimizing disruption.
-
Budget-conscious solutions scaled precisely to current and projected risks and growth.
As threats continue advancing, AI-powered custom security promises resilient and evolving protection without one-size-fits-all limitations.
How is AI being used in cybersecurity?
Artificial intelligence is revolutionizing cybersecurity in several key ways. Here are three of the most impactful applications of AI in protecting systems and data:
Automated Threat Detection
AI algorithms can process massive streams of system and network activity data to identify anomalies and detect potential cyber threats in real time. By establishing baselines of normal behavior, AI can quickly flag unauthorized access attempts, malware infections, or other suspicious activity for human analysts to investigate. This allows security teams to respond faster to emerging attacks.
Fraud Prevention
AI tools can analyze large volumes of transactions and customer interactions to detect patterns of fraudulent behavior not easily spotted by humans. Whether it’s phishing websites, stolen payment cards, or account takeover attacks, machine learning helps identify fraud faster and more accurately. This protects companies and customers alike.
Vulnerability Management
Applying AI to vulnerability scanning and penetration testing helps ensure organizations fix security flaws before attackers can exploit them. By automatically classifying vulnerabilities by risk level, AI makes it easier for admins to patch critical issues promptly. Continuous vulnerability monitoring with AI also allows the identification of misconfigured systems or apps over time.
While AI introduces its own risks, such as bias or accuracy concerns, it remains one of the most promising technologies for transforming cybersecurity defenses. With thoughtful implementation tailored to each organization’s needs, AI can enable security teams to lock down critical systems far more effectively amidst a threat landscape that grows more complex every day.
What is the AI trend in cybersecurity?
Cybersecurity threats are rapidly evolving, with hackers using increasingly sophisticated techniques to breach systems. Artificial intelligence (AI) offers new ways for security teams to stay one step ahead.
AI tools can analyze massive amounts of data to detect anomalies and identify threats faster than humans alone. They also continuously learn and improve to adapt to new attack methods.
Some key trends in using AI for cybersecurity include:
-
Automated threat detection – AI algorithms automatically scan network activity logs, endpoint behaviors, cloud workloads and more to flag anomalies in real-time, freeing up security analysts to focus on critical incidents. Top vendors like Darktrace and Vectra specialize in self-learning network/endpoint detection and response.
-
Fraud prevention – AI analyzes each online transaction to determine legitimacy, blocking fraudulent attempts before financial or data loss occurs. Features like user behavior modeling and real-time risk scoring enable adaptive protection.
-
Insider threat detection – By creating employee profiles combining HR data, psychometric assessments and dynamic behavior analysis, AI solutions can detect employees acting unusually or exhibiting risky warning signs. Features like automated user activity monitoring and privilege abuse alerts help security teams intervene early.
-
Intelligent orchestration of tools – AI "security orchestration, automation and response" (SOAR) platforms combine the feeds from all existing tools into one dashboard. This enables faster incident response, auto-remediation of common threats, and optimized workflows.
With exponentially increasing data volumes and attack sophistication, AI is becoming essential for effective cyber defense. Custom integrations ensure each organization harnesses AI aligned to their unique infrastructure, regulations and risk appetite.
What is the main challenge of using AI in cybersecurity?
AI brings promise for enhancing cybersecurity, but also introduces unique challenges that must be addressed.
One of the primary challenges is defending against deepfake attacks. Deepfake technology uses AI to create synthetic media like fake videos, images, and audio recordings that appear authentic. Attackers can leverage deepfakes to impersonate individuals or organizations, tricking victims into divulging sensitive information or taking harmful actions.
For example, a cybercriminal could use deepfake technology to imitate a company’s CEO in a video call. The attacker asks an employee to wire funds to an account for an "urgent business need." Without realizing the video is a deepfake, the employee complies, resulting in stolen funds.
Defending against these realistic-looking synthetic attacks requires advanced AI detection methods. Traditional cybersecurity tools often fail to identify manipulated media. AI models must be trained on diverse deepfake datasets to effectively spot anomalies in things like facial movements, speech patterns and inconsistencies across video frames.
However, deepfake generation technology evolves rapidly, allowing attackers to evade defenses over time. Ongoing research into more robust deepfake detection is critical. AI in cybersecurity must evolve alongside emerging generative AI threats.
In summary, deepfake attacks present a complex challenge for AI security solutions. Customized AI models are needed to identify manipulated media, but models require constant updating as attack methods advance. Specialized expertise in adversarial AI is key to developing reliable safeguards against this growing threat.
How AI is changing the cybersecurity landscape?
Artificial intelligence is rapidly transforming cybersecurity, providing new ways to protect systems from evolving threats. AI tools can analyze massive amounts of data to detect anomalies and identify breaches faster than human analysts. Additionally, AI algorithms can adapt in real-time to new attack methods, allowing defenses to stay one step ahead.
Here are some key ways AI is improving cybersecurity:
Accelerated Threat Detection
- AI models can process billions of security events per day and identify threats missed by rules-based systems. This enables earlier detection of sophisticated attacks.
Automated Response Capabilities
- Once threats are detected, AI systems can instantly execute countermeasures to neutralize attacks and prevent data loss. This eliminates reliance on manual processes.
Proactive Protection
- By detecting patterns in malware code and hacker behaviors, AI can predict future moves and recommend preventative safeguards before damage occurs.
Risk-based Resource Allocation
- AI tools provide visibility into an organization’s unique weak points, allowing security teams to focus efforts on high-risk vulnerabilities rather than spreading resources thinly.
While AI introduces risks if compromised, its ability to rapidly adapt makes cyberdefenses stronger overall. With customized AI solutions, companies can tackle their unique security challenges more effectively.
sbb-itb-178b8fe
Identifying Unique Security Challenges for AI Solutions
AI solutions for cybersecurity can be customized to address the specific threats and vulnerabilities faced by different organizations. By analyzing an organization’s assets, data flows, and risk landscape, AI tools can be tailored to monitor, detect, and respond to threats in a targeted way.
Case-by-Case: AI in Cybersecurity Examples
For example, a financial services company may be concerned about data exfiltration and insider threats, while a healthcare provider prioritizes protecting patient data privacy. AI systems can be designed to focus on detecting anomalies in financial transactions or access logs based on the organization’s concerns.
Similarly, an e-commerce site may utilize AI to spot payment fraud in real-time, while a cloud services provider could leverage AI to quickly identify misconfigurations that could lead to data leaks. The flexibility of AI allows for case-by-case customization.
Analyzing Risks and Tailoring AI Responses
Security teams can conduct in-depth analysis of an organization’s surface area, data flows, compliance needs, and threat models. This allows them to pinpoint specific cyber risks to address.
AI engineers can then design and train systems to monitor for threats related to those high priority risks. For example, user behavior analytics focused on spotting insiders accessing unauthorized data. Or network traffic analysis hunting for exploit attempts on vulnerable applications.
Balancing Security Needs with AI Capabilities
When mapping security needs to AI solutions, the capabilities of AI systems must align with the organization’s requirements. AI excels at tasks like pattern recognition and anomaly detection. But organizations may also need human-driven processes.
By balancing AI with policies, access controls, employee training, and incident response procedures, organizations can build defense-in-depth. AI enhances rather than replaces other critical security measures for a customized and resilient posture.
Developing AI in Cybersecurity Projects
AI has immense potential to transform cybersecurity, but developing effective AI systems requires thoughtful planning and execution. Here are key steps for initiating AI cybersecurity projects, building core components, and overcoming common challenges.
Initiating AI Cyber Security Projects
When launching an AI cybersecurity initiative, first define the specific security objectives and outcomes sought. Gather business requirements and data to determine where AI could make an impact. Assess existing infrastructure and workflows to identify opportunities for AI augmentation. Outline project goals, success metrics, milestones, and responsibilities across teams. Allocate budget for data infrastructure, AI platforms, development, and testing.
Consider starting with a limited pilot focused on one security domain before expanding AI capabilities more broadly. This focused approach allows for rapid iteration and learning.
Key Components of AI Cybersecurity Systems
AI cybersecurity systems typically comprise data pipelines, algorithms, and deployment mechanisms.
Data Infrastructure: High-quality, well-organized data fuels AI. Establishing data pipelines from various security tools and sources is essential. Carefully preprocess and label datasets for algorithm training.
Algorithms: Choose AI techniques like anomaly detection, natural language processing, or computer vision based on the use case. Leverage transfer learning from open-source models when applicable. Fine-tune models on representative, high-quality data samples.
Deployment System: Wrap models into user-friendly applications with monitoring, explainability, and feedback loops. Integrate via APIs with existing security infrastructure. Containerize components for portability across environments.
Challenges and Solutions in AI Project Development
Data Complexity: Connecting data silos is challenging. Applying MLOps and gradual data centralization helps structure workflows. Building internal tools to synthesize and label security data takes sustained effort but enables better algorithm training.
Model Interpretability: It’s often unclear why AI models make certain decisions, which hampers trust and adoption. Explainable AI techniques help decipher model logic and illuminate limitations. Ongoing human-in-the-loop evaluation provides oversight and guards against unexpected errors.
With thoughtful design and execution, AI cybersecurity initiatives can make systems more adaptive, accurate, and scalable. But integrating AI is an iterative process requiring collaboration across security, data, and engineering teams.
Integrating AI Tools into Existing Cybersecurity Frameworks
Cybersecurity teams can strengthen their defenses by incorporating AI tools into current systems. With thoughtful integration, AI can enhance legacy frameworks rather than replace them.
The Synergy between AI and Legacy Systems
AI excels at processing large datasets and detecting subtle patterns. By combining these capabilities with existing rule-based systems, organizations benefit from both traditional and modern techniques.
Here are some examples of effective integration:
- Using AI to analyze network traffic data and identify new threats missed by signature-based tools
- Employing natural language AI to scan documents and communications for signs of social engineering
- Automating the analysis of security logs and events to quickly surface high-risk incidents
With planning and testing, AI tools can work alongside longtime systems to boost overall performance.
Interoperability Considerations for AI Tools
To enable a smooth integration process, IT leaders should evaluate new AI systems using criteria such as:
- Data formats: Can the AI tool ingest logs, alerts, and other data from current security platforms? Solutions that support common formats like JSON and log file standards can more easily connect.
- API availability: Does the AI platform offer APIs for linking into existing architectures? API access enables direct connections.
- Tool flexibility: Can the AI be customized at the model and interface levels to meet specific integration needs? More adjustable systems can better fit alongside legacy tools.
Additionally, rolling out AI incrementally and monitoring its impact can prevent disruption.
Case Study: A Successful Integration Story
When Hartford Healthcare sought to enhance its cybersecurity strategy, it turned to AI to bolster existing systems from vendors like Palo Alto Networks and Fortinet.
The healthcare provider deployed Vectra AI’s platform to detect intrusions missed by other tools. With robust APIs, Vectra seamlessly integrated into Hartford’s security infrastructure.
Now, the Vectra AI analyzes network metadata in real time, identifying behavioral anomalies suggestive of ransomware and other emerging attacks. By automatically integrating with existing incident response systems, Vectra allows Hartford’s security team to rapidly investigate and shut down threats.
Since the deployment, Vectra has consistently detected cyberattacks in early stages before major damage was done. This integration of legacy security tools with AI has reduced risk and strengthened Hartford’s defenses.
Artificial Intelligence in Cyber Security Research and Development
Artificial intelligence (AI) is transforming cybersecurity in profound ways. As cyber threats become more sophisticated, organizations require intelligent systems that can adapt and respond in real-time. Recent research developments demonstrate AI’s immense potential to bolster cyber defenses through advanced threat detection, automated response protocols, and predictive analytics.
Cutting-Edge Innovations in AI and Cybersecurity
Leading cybersecurity researchers have made breakthroughs applying deep learning and neural networks for cyber defense. For example, AI models can now:
- Analyze network activity and endpoints to identify sophisticated malware and zero-day attacks
- Correlate threat intelligence feeds with internal telemetry data to prioritize vulnerabilities
- Continuously monitor authentication systems and access controls for anomalies
- Generate customized threat hunting rules tailored to an organization’s tech stack
These AI cybersecurity use cases leverage supervised, unsupervised, and reinforcement learning techniques for enhanced threat visibility, faster response times, and more.
From Research to Reality: Translating Theory into Practice
While AI cybersecurity research shows immense promise, real-world deployment comes with unique challenges. Organizations must carefully evaluate their infrastructure, processes, and data flows to determine where and how to implement AI.
With the right strategy, businesses can operationalize leading-edge research to:
- Augment overburdened security teams with automated assistants
- Apply deep learning models to high-value data sources
- Maintain optimal AI model performance through continuous tuning
- Ensure transparency and auditability of AI systems
The Future of AI in Cybersecurity: Trends and Predictions
Experts forecast AI will soon become integral to cybersecurity stacks. Key trends include:
- Real-time, AI-powered threat hunting and response
- Granular identity and access implementations with AI
- Extensive use of NLP and chatbots for security workflows
- Widespread adoption of AI-generated threat models
As research translates into commercial solutions, organizations must invest today to realize AI’s benefits. With diligent strategy planning guided by internal user needs, businesses can harness AI’s power for enhanced cyber resilience.
Conclusion: The Paradigm Shift of AI in Cybersecurity
Revisiting the Impact of AI on Cybersecurity
AI is transforming cybersecurity in significant ways. By automating threat detection and response, AI enables security teams to act faster and more efficiently against attacks. AI also provides predictive capabilities to identify vulnerabilities and anomalies that could lead to breaches.
Additionally, AI helps address the cybersecurity skills shortage by augmenting existing staff. It allows organizations to make the most of their limited security resources.
Overall, AI is driving better protection of critical systems and data from bad actors seeking to infiltrate networks or steal information.
The Road Ahead for AI and Cybersecurity Integration
As threats continue to evolve, integrating AI-powered solutions into cybersecurity frameworks will only increase in importance. Organizations that embrace custom AI will stand the best chance of securing their digital assets.
With the right expertise and careful planning around use cases, AI can provide robust defenses tailored to each company’s unique needs. As innovation in the field continues, more advanced AI capabilities will emerge to counter sophisticated hacking techniques.
The future looks bright for this synergistic relationship between AI and cybersecurity. Together, they can enable organizations to operate confidently as they digitally transform.
Related posts
- AI and Automation: Navigating the Future of Efficiency
- AI Process Automation: The Competitive Advantage
- AI for Education: Personalizing the Learning Experience
- AI for Retail Automation